Using AI to predict new materials with desired properties
- 2020年08月01日 06:00:00
- テクノロジー
- JCN Newswire
- コメント
Aluminum alloys are lightweight, energy-saving materials made predominantly from aluminum, but also contain other elements, such as magnesium, manganese, silicon, zinc and copper. The combination of elements and manufacturing process determines how resilient the alloys are to various stresses. For example, 5000 series aluminum alloys contain magnesium and several other elements and are used as a welding material in buildings, cars, and pressurized vessels. 7000 series aluminum alloys contain zinc, and usually magnesium and copper, and are most commonly used in bicycle frames.
Experimenting with various combinations of elements and manufacturing processes to fabricate aluminum alloys is time-consuming and expensive. To overcome this, Ryo Tamura and colleagues at Japan's National Institute for Materials Science and Toyota Motor Corporation developed a materials informatics technique that feeds known data from aluminum alloy databases into a machine learning model. This trains the model to understand relationships between alloys'mechanical properties and the different elements they are made of, as well as the type of heat treatment applied during manufacturing. Once the model is provided enough data, it can then predict what is required to manufacture a new alloy with specific mechanical properties. All this without the need for input or supervision from a human.
The model found, for example, 5000 series aluminum alloys that are highly resistant to stress and deformation can be made by increasing the manganese and magnesium content and reducing the aluminum content. "This sort of information could be useful for developing new materials, including alloys, that meet the needs of industry,"says Tamura.
The model employs a statistical method, called Markov chain Monte Carlo, which uses algorithms to obtain information and then represent the results in graphs that facilitate the visualization of how the different variables relate. The machine learning approach can be made more reliable by inputting a larger dataset during the training process.
Further information
Ryo Tamura
National Institute for Materials Science
tamura.ryo@nims.go.jp
Paper: https://doi.org/10.1080/14686996.2020.1791676
About Science and Technology of Advanced Materials Journal
Open access journal STAM publishes outstanding research articles across all aspects of materials science, including functional and structural materials, theoretical analyses, and properties of materials.
Chikashi Nishimura
STAM Publishing Director
NISHIMURA.Chikashi@nims.go.jp
Press release distributed by ResearchSEA for Science and Technology of Advanced Materials.
Copyright 2020 JCN Newswire. All rights reserved. www.jcnnewswire.com
怪物右腕のガールフレンド、バミューダ島での水着特集撮影を公開 超セクシー水着でポーズ決める
グルメ王・渡部建が告白、食レポの極意「これやってるヤツいない、渡部去った後」44歳芸人も納得
新名神逆走事故 容疑者「道に迷った」と供述 本線上でUターンか
【巨人】エース戸郷翔征が勝てない 開幕から6戦連続未勝利
浜辺美波「25歳の今夏は」… 背中が開いた鮮やか赤色衣装の振り返りショットで報告
海南航空、大阪/関西〜深セン線を運休 5月8日から
超無課金こと石田拳智氏が1100万円寄付「地元のためなら」彦根市の無痛MRI乳がん検診に
【阪神】ビーズリー、34日ぶり1軍先発へ「自分の中では何も変えず」4月17日ヤクルト戦以来
【阪神】岡田彰布顧問「お客さんがやかましいから打たなかったんですよ」伝説の3連発秘話明かす
容疑者「注意され、家族全員殺そうと思った」 千葉立てこもり
父が再婚の丸山隆平(36)現在の家族関係がとんでもないことになっていたと話題に
後藤真希、“衝撃を受けたアイドル”を聞かれズバリ実名告白「誰もが衝撃を受けたと思う」
二階堂ふみが結婚!?お相手が衝撃的過ぎてネット民「マジか・・・」
中居正広氏側の反論の6文字に違和感「誰の言葉?」「とても引っかかる」X議論白熱/送付全文
「80歳に見えない」国民的女優、のんと2ショット「若い頃に似てる」「奇跡」圧倒的美貌に騒然
5人組アイドルBrainBeatにーなさん死去「不慮の事故により永眠いたしました」公式X
和田アキ子突如泣き出した 番組で異例の「トイレ休憩」後にハプニング スタジオ騒然
指原莉乃「実はすんごいことが起きまして」喜びの報告に「本当に凄い」「私まで嬉しい」祝福の声
柏木由紀に「流出した写真でエライ事に」芸人の“プチ炎上”ツッコミに釈明「今、まっとうに」
多部未華子(30)結婚の裏事情あまりにも恐ろしすぎると話題に!
父が再婚の丸山隆平(36)現在の家族関係がとんでもないことになっていたと話題に
二階堂ふみが結婚!?お相手が衝撃的過ぎてネット民「マジか・・・」
後藤真希、“衝撃を受けたアイドル”を聞かれズバリ実名告白「誰もが衝撃を受けたと思う」
小澤征悦と再婚した桑子真帆アナ(34)黒い過去が流出、衝撃の過去にネット騒然
多部未華子(30)結婚の裏事情あまりにも恐ろしすぎると話題に!
関ジャニ錦戸亮、登場人物が全員クズだらけの不倫劇もメディア沈黙で批判殺到
「名探偵コナン」最大の謎、蘭姉ちゃんのあの角の正体がついに判明
ガーシー、佐野ひなこの暴露を示唆でネット騒然「ファンだったのに」
中居正広氏側の反論から浮かんだ2つの新事実を指摘「性暴力の評価はともかく…」紀藤正樹弁護士
【おすすめアニメ50選】完結済み!定番から最新作まで!
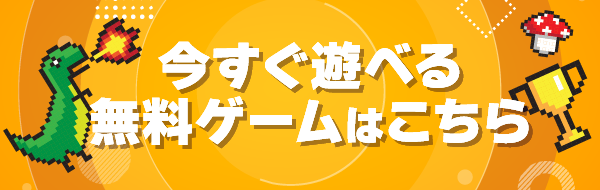
怪物右腕のガールフレンド、バミューダ島での水着特集撮影を公開 超セクシー水着でポーズ決める
グルメ王・渡部建が告白、食レポの極意「これやってるヤツいない、渡部去った後」44歳芸人も納得
新名神逆走事故 容疑者「道に迷った」と供述 本線上でUターンか
【巨人】エース戸郷翔征が勝てない 開幕から6戦連続未勝利
浜辺美波「25歳の今夏は」… 背中が開いた鮮やか赤色衣装の振り返りショットで報告
海南航空、大阪/関西〜深セン線を運休 5月8日から
超無課金こと石田拳智氏が1100万円寄付「地元のためなら」彦根市の無痛MRI乳がん検診に
【阪神】ビーズリー、34日ぶり1軍先発へ「自分の中では何も変えず」4月17日ヤクルト戦以来
【阪神】岡田彰布顧問「お客さんがやかましいから打たなかったんですよ」伝説の3連発秘話明かす
夏の夜に美髪&リラックス、「いち髪」より新ヘアケア限定発売